ITB Researchers Develop IoT for Optimization of Hydroponic Agriculture
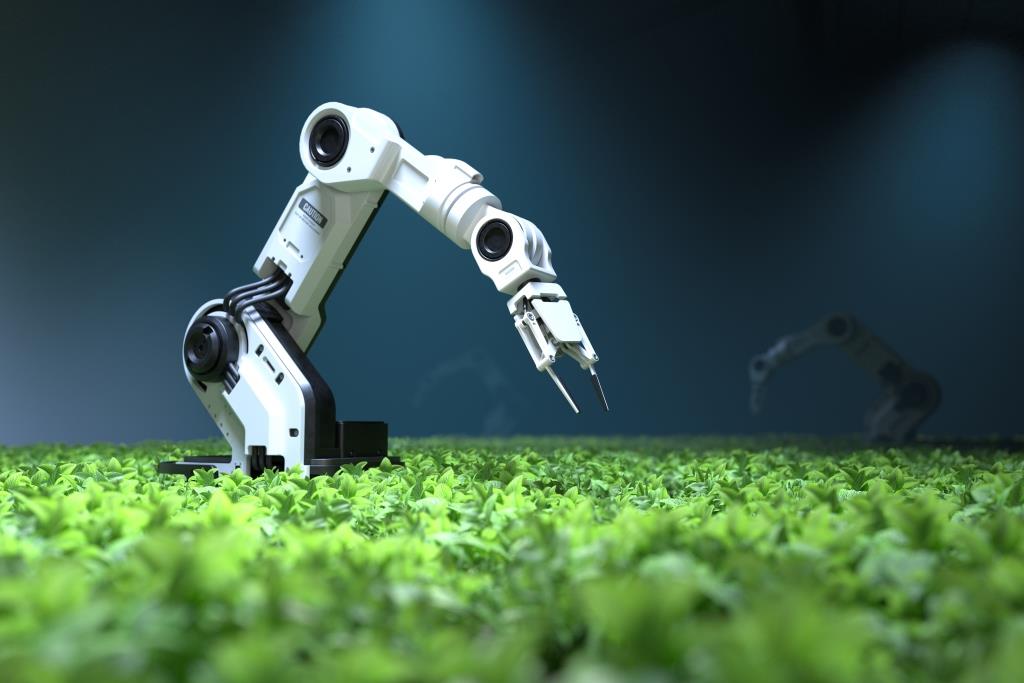
*Photo illustration. Source: freepik
BANDUNG, itb.ac.id—One of the consequences of the drastic global population growth is the rising demand for food. Current food supplies could not keep up with the demands due to most agricultural grounds being converted into settlements. However, hydroponic technology may be a viable solution to the declining availability of agricultural land.
The benefits of this technology are less use of space and an increase in harvest. Therefore, the research team consists of Maman Budiman, Ph.D. (Physics Instrumentation and Computation Research Group, FMNS ITB), Dr. Nina Siti Aminah (Physics Instrumentation and Computation Research Group, FMNS ITB), Ant. Ardath Kristi, S.T. (Indonesian Institute of Sciences), Efraim Partogi and Prianka Anggara (students of Ant. Ardath), designed a prototype of an IoT-based (Internet-of-Things) instrumentation system for hydroponic agriculture.
The instrumentation system can monitor physical parameters of plant growth to determine and regulate their influences on hydroponics production. ML (Machine Learning) technology is also implemented to predict the production yield, using algorithms of random forest, linear, and polynomial regressions.
Quoted from the LPPM ITB site, the cultivated plants- bok choy (Brassica rapa subsp. Chinensis) and water spinach (Ipomoea aquatica)- were studied using the NFT (Nutrient Film Technique) hydroponic system.
"This research was conducted on the plants grown at 'Blessing Farm', West Bandung, West Java. The light intensity given to the plants is controlled by the roof. Moreover, the nutrition system for the entire vegetation relies on one nutrient tank," the research team explained.
This article has been published in the Engineering Rubric of ITB Media Indonesia. The full article can be accessed via the following link: https://research.lppm.itb.ac.id/information/hidroponik_komersial_berbasis_internet_of_things
To monitor the physical parameters, various sensors and components are equipped with the instrumentation system. All sensors are connected by a microcontroller, each with a wi-fi module for linking to the server. The data obtained is processed, stored in a database, and divided into two types: training and testing data. Training data create prediction models, which are tested for their performance using the testing data. If the performance level of the model contains additional data or does not reach the criteria, the data will undergo the retraining process until the acquired model satisfy all the performance standards.
From data of atmospheric and solution temperature, humidity, light intensity, and TDS (Total Dissolved Solids) observed as independent variables, the plant height, as well as its number and area of leaves, were obtained as predicted dependent variables.
The highest coefficient value of determination for predicting the best bok choy production was 0,933, as calculated from the random forest regression algorithm. It was revealed from the independent variable data of bok choy and water spinach production that the most influential growth parameters are TDS and light intensity. These two can be set as the controlling variable, in which the control system for TDS will be made from several models of the random forest regression program. Hence, these plants can grow optimally in various weathers to produce the highest quality of leaves.
Reporter: Najma Shafiya (Postharvest Technology, 2020)
Translator: Ruth Nathania (Environmental Engineering, 2019)
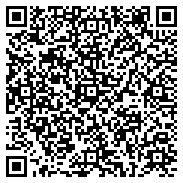
scan for download