The Implementation of Data Science in Agriculture
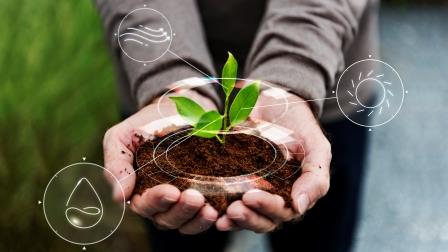
BANDUNG, itb.ac.id–Data science is an applied science that focuses on analyzing and studying how to extract information from existing data sets. Dr. Juro Miyasaka of Kyoto University was invited as a speaker in the Visiting Professor Program: International Guest Lecture on Wednesday (27/10/2021) by the Agrotechnology and Bioproduct Technology Research Group (KK ATB) of School of Life Sciences and Technology ITB.
The lecture was titled "Basics of Data Science and Applications to Agricultural Production." This presentation began by providing an understanding of the standard process in data mining, such as the cross-industry standard process for data mining, or CRISP-DM, as it is more commonly abbreviated.
"In general, CRISP-DM is used for data mining in the business sector, which has an early stage of business understanding. When it comes to agricultural applications, the first step is to understand agricultural production itself," said Dr. Juro Miyasaka. He went on to say that data science can be used for machine learning, both supervised and unsupervised learning, to help the agricultural sector grow.
Linear regression is another application of data science methods. This method is commonly used to predict the amount based on existing data by training the model in advance, such as predicting rice production based on data from several years before.
"Creating a model from existing data is easier, but we can't guarantee an optimal result," he explained. Dr. Juro then reminded everyone that the overfitting event must be carefully considered during the model training stage; when the data used to train the model is only the best, the accuracy is very low when tests are performed using different data.
According to Dr. Juro, the use of predictive models based on data sets in agriculture can be used to predict the possibility of a disease. In the case of Coleosporium plectranthus Barclay disease on Perilla plants, the data that must be collected before developing a predictive model include the plant's temperature and humidity, the temperature of the growing media, and the plant's height. Data obtained from manual or sensor data collection that is completed within 10 minutes. "The support vector machine and random forest methods are used to predict disease in this plant," he explained.
Another example given in his presentation was the prediction of Mizuna plant growth rate using environmental data. In contrast to the disease prediction model on Perilla plants, the growth rate is predicted using correlation and Adaboost k-nearest neighbor. The data collected is also much more complex, including the average temperature and humidity of the growing media, the average environmental temperature, the average ambient temperature at night, the average concentration of carbon dioxide in the environment, and the average humidity of the environment. This information is also derived from various plant height measurements.
Dr. Juro Miyasaka concluded by emphasizing that methods in the field of data science have yet to be explored and applied to agricultural production.
Reporter: Athira Syifa P. S. (Post-harvest Technology, 2019)
Translator: Naffisa Adyan Fekranie (Oceanography, 2019)
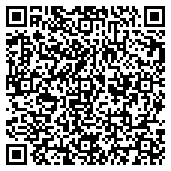
scan for download